The world of artificial intelligence is undergoing a seismic shift with the rise of multimodal AI systems. These advanced models are breaking barriers by integrating and processing diverse forms of data—images, text, videos, and even physical actions—into a single, cohesive framework. From robotics and virtual assistants to user interface (UI) automation, these AI agents are transforming how machines interpret and act on complex inputs, enabling them to perform tasks in both digital and physical environments. By merging verbal and spatial intelligence through deep learning, these systems are redefining the way humans and machines interact.
But as groundbreaking as these advancements are, they come with their own set of challenges. Enter Magma, a revolutionary multimodal AI model developed by Microsoft Research in collaboration with leading academic institutions. Magma is designed to unify vision, language, and action, offering unprecedented flexibility and adaptability for a wide range of real-world applications. Let’s dive into what makes Magma a game-changer in the AI landscape.
The Challenge of Multimodal Integration
While AI models have made significant strides in specialized domains—such as vision-language understanding or robotic manipulation—they often struggle to combine these capabilities into a single, unified system. Most existing models are tailored for narrow, domain-specific tasks, like navigating digital user interfaces or manipulating physical objects. This specialization limits their ability to adapt to different environments and perform complex, real-world tasks that require both spatial and temporal reasoning.
For example, a model trained to navigate UIs might excel in digital environments but fail to translate those skills to physical robotics. Similarly, a robot trained for object manipulation might struggle with tasks that require understanding textual or visual cues. Traditional Vision-Language-Action (VLA) models attempt to bridge this gap by pretraining on large datasets of vision-language pairs and action trajectories. However, these models often lack the flexibility to operate effectively in both structured digital and unstructured physical environments.
This is where Magma steps in, offering a unified solution that overcomes these limitations.
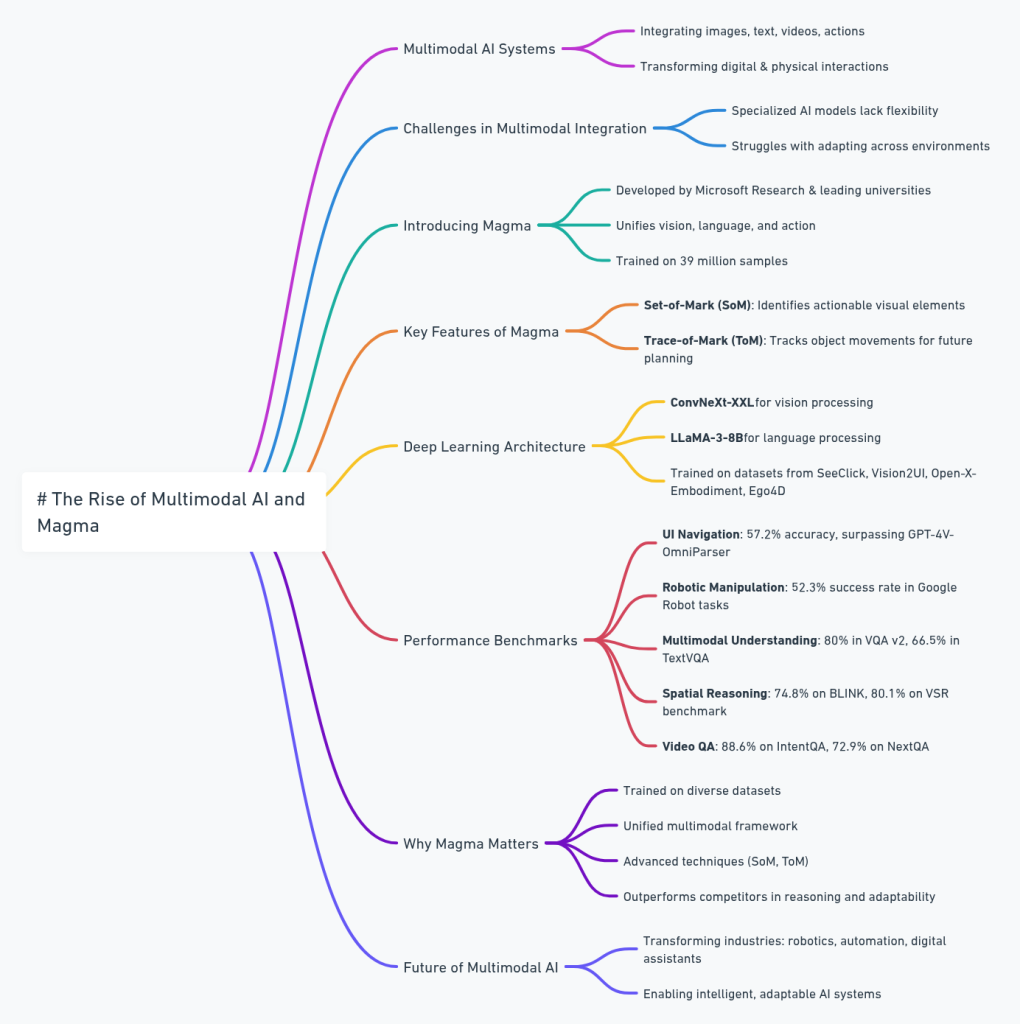
Introducing Magma: A Unified Multimodal AI Model
Developed by researchers from Microsoft Research, the University of Maryland, the University of Wisconsin-Madison, KAIST, and the University of Washington, Magma is a cutting-edge foundation model designed to seamlessly integrate multimodal understanding and action execution. By combining vision, language, and action into a single framework, Magma enables AI agents to operate across both digital and physical domains with remarkable adaptability.
Magma’s training methodology is as impressive as its capabilities. The model was trained on a massive and diverse dataset comprising 39 million samples, including:
- 2.7 million UI screenshots for digital navigation tasks
- 970,000 robotic action trajectories for physical manipulation
- 25 million video samples for temporal and spatial reasoning
This expansive dataset allows Magma to perform a wide range of tasks, from navigating user interfaces to manipulating objects in the physical world. But what truly sets Magma apart are two innovative techniques:
- Set-of-Mark (SoM): This technique enables Magma to identify and label actionable visual objects, such as buttons and clickable elements in UI environments. By providing a more accurate understanding of digital contexts, SoM enhances Magma’s ability to navigate complex interfaces.
- Trace-of-Mark (ToM): ToM allows Magma to track object movements over time, improving its ability to anticipate and plan future actions in dynamic physical environments.
Together, these techniques empower Magma to not only process and understand multimodal data but also execute actions based on that understanding.
A Deep Learning Architecture Built for Multimodal Mastery
Magma’s architecture combines state-of-the-art deep learning models for vision and language processing. The model leverages a ConvNeXt-XXL vision backbone to process images and videos, paired with the LLaMA-3-8B language model to handle textual inputs. This powerful combination allows Magma to integrate vision-language understanding with actionable intelligence, enabling it to navigate user interfaces and manipulate objects in the physical world with remarkable precision.
To ensure robust performance across diverse applications, Magma was trained on a variety of datasets, including:
- UI navigation tasks from SeeClick and Vision2UI
- Robotic manipulation datasets from Open-X-Embodiment
- Instructional videos from Ego4D, Something-Something V2, and Epic-Kitchen
This comprehensive training approach ensures that Magma can generalize across different modalities, making it a highly versatile AI agent.
Performance Results: Magma Outshines the Competition
Magma has demonstrated exceptional performance across a range of benchmarks, outperforming existing multimodal AI models in several key areas:
- UI Navigation: Magma achieved a 57.2% element selection accuracy in zero-shot UI navigation tasks, surpassing models like GPT-4V-OmniParser and SeeClick.
- Robotic Manipulation: In robotic manipulation tasks, Magma achieved a 52.3% success rate in Google Robot tasks and a 35.4% success rate in Bridge simulations, significantly outperforming OpenVLA.
- Multimodal Understanding: Magma scored 80.0% accuracy in VQA v2, 66.5% accuracy in TextVQA, and 87.4% accuracy in POPE evaluations.
- Spatial Reasoning: The model demonstrated strong spatial reasoning abilities, scoring 74.8% on the BLINK dataset and 80.1% on the Visual Spatial Reasoning (VSR) benchmark.
- Video Question-Answering: Magma achieved 88.6% accuracy on IntentQA and 72.9% accuracy on NextQA, showcasing its ability to handle temporal reasoning effectively.
These results highlight Magma’s ability to not only understand multimodal inputs but also execute actions in both digital and physical domains. What’s more, Magma achieves this without requiring additional fine-tuning, making it an exceptionally adaptable AI agent.
Key Takeaways: Why Magma Matters
- Training on Diverse Datasets: With 39 million multimodal samples, Magma is equipped to handle a wide range of tasks, from UI navigation to robotic manipulation.
- Unified Framework: By combining vision, language, and action into a single model, Magma overcomes the limitations of domain-specific AI systems.
- Advanced Techniques: SoM and ToM enhance Magma’s ability to label objects and plan long-term actions, significantly boosting its performance.
- Impressive Performance Gains: Magma outperformed existing models by 19.6% in spatial reasoning benchmarks and improved by 28% in video-based reasoning tasks.
- High Adaptability: Magma’s ability to generalize across multiple domains without additional fine-tuning makes it a versatile solution for various industries.
- Industry Impact: From robotics and autonomous systems to UI automation and digital assistants, Magma’s capabilities are set to revolutionize decision-making and execution across industries.
The Future of Multimodal AI
Magma represents a significant leap forward in the field of multimodal AI. By bridging the gap between vision, language, and action, it sets a new standard for intelligent, autonomous systems capable of operating seamlessly across diverse environments. As AI continues to evolve, models like Magma will play a pivotal role in shaping the future of human-machine interaction, paving the way for smarter, more adaptable technologies that can tackle the complexities of the real world.
Whether it’s navigating a digital interface, manipulating objects in a physical space, or making intelligent decisions based on multimodal inputs, Magma is poised to transform industries and redefine what’s possible with AI. The future is here—and it’s multimodal.